AI agents are hyped as the future of automation, set to transform workflows and drive efficiency. But beyond the buzz, enterprise adoption is still in its early days. Together with our friends from Endeit, we’ve dug deep - speaking with industry leaders, AI builders, and emerging players to cut through the noise. Who’s leading the charge, and what’s holding AI agents back from true breakout growth?This is Part I of our deep-dive series - starting with a market reality check before exploring real-world applications and implications for founders.
Part I: AI Agents – Market Trends & VC Observations
AI agents, the race is on
Imagine a world where customer service never sleeps, financial advice is always on point and logistics run like clockwork – all powered by smart, automated systems without you lifting a finger. Sounds like a futuristic fugazi? Yet, more and more start-ups (claim to) build exactly that: digital companions taking over cumbersome work, freeing up time for more creative, value-creating tasks.
Just like many other emerging technologies, AI agents raise great expectations, driven by their potential to transform work as we know it today - creating FOMO among organizations and VCs alike. Hence, the race to capitalize on AI agents is on.
For us as VCs, the rapid AI agent buzz implies massive enterprise value creation potential. And yet, we also observe that AI agents generate tremendous hype, thereby driving investment decisions of VCs and organisations alike fueled by FOMO/excitement rather than solid judgement. If history has taught us one thing, it is that new technology rarely unfolds as expected. Just think of e.g. the dot-com bubble (as -still- often cited by our senior partners) or more recently the metaverse- and crypto crash.... That is why we at Endeit and Capnamic have joined forces to demystify AI agents, separate signals from noise and try to identify what in our mind make the most promising investment areas. To do so, we have spoken with founders, experts and AI practitioners—including Jan Andresen, David Asabina, Atte Honkasalo, Clemens Wasner, Alois Krtil and Sushel Bijganath. We explored key questions: How mature is the market? Which industries will adopt AI agents first? And what might differentiate the winners?
Let’s start with the basics: AI agents - A practical definition
Short and sweet: There is no universal definition of an AI agent - and we will not derive one either.
Rather, we believe that their design varies widely depending on technical specifics and the requirements of individual use cases. However, here is a practical analogy: think of an AI agent as a new hire. When onboarding a graduate, she comes equipped with either some general or domain-specific knowledge. Yet, before she can work autonomously you have to set clear objectives, provide step-by-step training, the necessary tooling and teach her how her work fits into the bigger picture and the limitations of the function or job (like guardrails) #Purpose. Similarly, an AI agent is a large language model (LLM) enhanced with tools like APIs, Natural Language Processing (NLP) or other integrations, enabling it to "think" and "act" in an agentic way. This autonomous "tool use" is at the core of what makes AI agents distinct and agentic—they can ‘perceive’ their environment, reason, make decisions and act independently to achieve predefined outcomes. While a standalone LLM can ‘only’ read and write. Adding tools and instructions transforms it into a dynamic system capable of solving complex, unstructured problems on its own, although the agent can only be “autonomous” within preset channels, data sets and human approvals at certain stages. Basically setting guardrails, like an autopilot in a plane.
It was developers completing these briefings, with platforms like crew.ai or n8n simplifying configurations by allowing them to use natural human language and low/no code aspects instead of traditional coding work. More and more non-technical users get empowered to drive automations. And then the vertical pre-set up AI agents arrived…
The Big Leap: Why This Tech is Game-Changing
But first things first: In order to understand the way forward, we need to go back first - process automation has come a long way, evolving from rule-following systems to intelligent, adaptable agents. Here is a breakdown of the three waves of automation we have been observing:
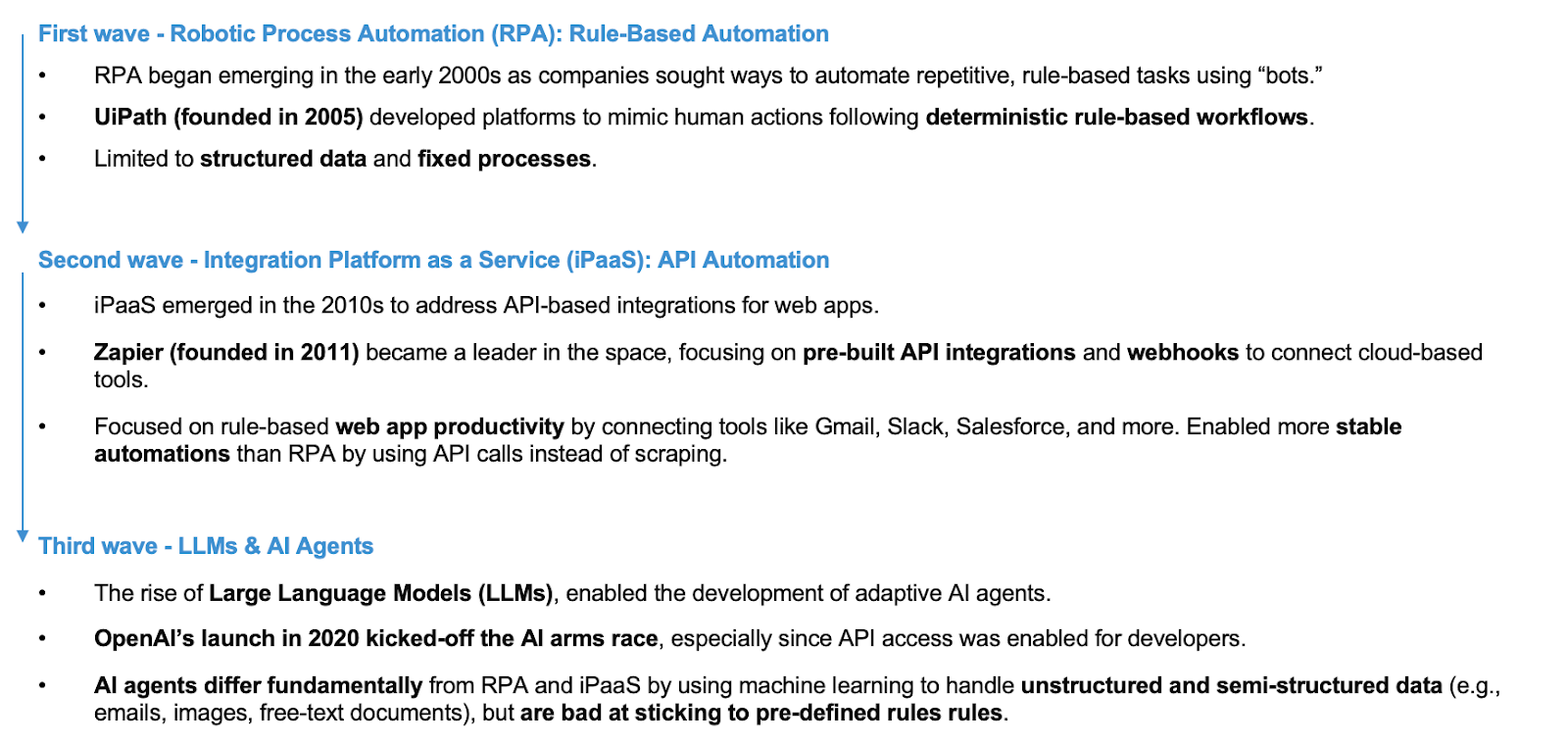
The First Wave: Rule-Based RPA – The Taskmaster
These tools are great at following explicit, step-by-step instructions set by developers but could not think for themselves or adapt to anything outside their programmed parameters. Platforms like UiPath became synonymous with this wave, mimicking human actions like copying and pasting data to perform repetitive tasks.
Example: Picture an RPA bot reconciling invoices—downloading files, matching line items and sending notifications. It is fast and efficient, but if even one invoice is in a different format, the bot throws up its virtual hands, requiring developers to step in to reprogram the rules.
RPA streamlined repetitive workflows but lacked flexibility, requiring constant developer oversight and struggling with unexpected scenarios. In large enterprises, running and maintaining RPA often required dedicated departments or Centers of Excellence with scarce, specialized expertise and significant budgets.
AI agents, on the other hand, promise a more flexible alternative, empowering also non-technical users to run and manage automations. Some argue that their ability to ‘think’ autonomously and be programmed using human language democratizes the setup and management of digital co-workers—at least in theory. In practice, however, AI agents do require well-designed architectures to deliver this desired outcome which is especially true for complex process structures.
The Second Wave: API-First Automation – The Connector
The second wave of tech brought us API-first architectures—essentially the glue that links platforms together through webhooks, creating seamless data flows 🔗. Over the past couple of years, the start-up scene has been flooded with vertical API-first softwares, all promising to connect existing tools and act as an extension of what is already out there. Many of these solutions delivered solid value, often addressing specific, niche problems. The poster child of this wave? Zapier, a horizontal platform for businesses looking to integrate systems and automate workflows.
Example: Think of a SaaS company automating customer onboarding. API-first systems pull data from a signup form, trigger a pre-written standardized welcome email and sync user information across CRM and billing systems. Everything runs smoothly—as long as the APIs stay intact and content is pre-scripted.
Like RPA, API-first systems were rule-based and prone to breaking when APIs or metadata is changed, requiring constant upkeep. New AI research is tackling this by enabling LLMs to autonomously select and adapt to tools, reducing versioning errors. Even though still in its infancy, projects like Toolformer and Gorilla are trying to make LLM tool-use of APIs more flexible and resilient or web scraping such as Airtop being just one example. This has the potential to change how companies think about building and scaling interconnected systems.
The Third Wave: Accessible LLMs – The Problem-Solver
Enter the third wave: automation powered by large language models (LLMs). Think of this as the leap from following scripts to solving puzzles. They are not just rule-followers—they are decision-makers capable of context-aware, dynamic actions.
Example: Imagine an AI agent acting as a customer support representative. It reads a customer email (unstructured data), interprets the issue, searches the knowledge base for answers and responds for every possible scenario with enough training.
The third wave is defined by its degree of freedom tackling decisions we once thought only humans could handle. Unlike RPA or API-first systems, AI agents can handle the unexpected, adapt on the fly and even “self-evaluate” their responses. While they are great for solving complex, unstructured problems, they are not ideal for tasks that require rigid adherence to strict rules—where RPA and APIs still dominate.
How we see it, these three waves of automation are not mutually exclusive. At their best, modern systems integrate elements of all three—combining the reliability of RPA, the connectivity in other systems of API-first architectures and the adaptability of AI agents.
A Market Built on Assumptions: The Reality vs. Expectation Gap
The AI agent market is buzzing with bold projections - soaring from $5.1B in 2024 to $47.1B by 2030 (CAGR 44.8%). But the devil is in the details: about 80% of AI investment still goes to infrastructure, leaving just 20% for applications like AI agents. Revenue data from major LLM providers confirms that AI agents are not yet fully embedded in workflows/the workforce.

For example, in 2024, OpenAI presumably made over 75% of its revenue from subscriptions like ChatGPT Plus, while only 25% came from API-based use cases like AI agents and automation. One reason for this imbalance stemmed from an initial limited developer access and a delayed API rollout. Anthropic, in contrast, has leaned more heavily on application-based revenue from the beginning, signaling a stronger push toward embedded AI products - but at a completely different scale in terms of absolute dollars spent.
Also, a look at corporate IT budgets does reveal a massive gap between AI’s potential and its actual enterprise adoption to date, meaning that AI start-ups in the application layer are barely scratching the surface of an untapped market: In the EU, IT budgets typically account for approximately 10% of a company's revenue, while AI spending represents just 5% of IT budgets. Applying this to SMEs - which make up the majority of businesses in the EU - a company generating €20M in revenue would allocate around €2M to IT. In this analogy, only about €100K would be dedicated to AI across various business functions. Actual AI investment remains limited, with most spending still coming from experimental budgets. See the illustrative table below for reference; figures may vary by sector and specific budget allocations.

This clearly demonstrates that AI is still in its early innings. A compelling parallel can be drawn to the internet adoption journey, where AI penetration is currently estimated at just 4% of its total potential—putting us at the very beginning of its growth curve.

As VCs, a key question we must consider is: What fundamental drivers will enable AI agents to reach an inflection point and achieve mass adoption in businesses - just as broadband, cloud computing and mobile devices did for the internet? Equally important, in which sectors will this adoption take off first for us to generate timely returns for our investors? With increasing investment in AI applications and new entrants like Deepseek (open-source) and Qwen (Alibaba) intensifying competition and driving down compute costs, the market dynamics are shifting. Yet, despite this momentum, significant roadblocks continue to hinder large-scale adoption.